In the world of social interactions, it becomes quite important for businesses to keep a track of how their brand is performing in the market to the likes of people. Hence, tapping into the emotions that your audience exhibits becomes important for every brand. This is where sentiment analysis can play a big role.
Sentiment Analysis utilizes NLP (Natural Language Processing), text analysis and biometrics to identify, extract and quantify the subjective information. Sentiment analysis is commonly used in customer-facing materials including reviews and survey replies, as well as internet and social media interactions.
This analysis is done in order to understand the customer and their social sentiment around a brand product or service. This in turn helps a brand placing their product better in front of their target audience.
For this case study, we bring forward a product G+ (supposed name) which is basically an app that allows people to book their park rides and entry to one of the biggest entertainment parks in the country. And, we are going to analyze the customer sentiments around this product.
So, let’s get diving!
Sentiment Analysis: Emotion Detection and Analysis at a Deeper Level
For this analysis, the team at Factspan extracted customer interactions from platforms like Twitter, LinkedIn etc. which is basically referred to as Data Scraping.
Problem Statement:
- Higher customer attrition rate due to issues on the app.
- Reduced Sales for the park rides via app.
- Decreasing accuracy of the recommendation engine.
Main Areas of Concern:
There were mainly three areas of concern that our Data Engineers found while working on this case. They are as follows:
- G+ application faced crashes when the traffic load was high on the app.
- Anyone booking the service at around 7 am in the morning was able to get the benefits making it difficult for other people waking up later. The application was not initially made with this mindset.
- The cost of the G+ product application was on a higher end.
Our Approach:
- Collect data from different social media, tweets, blogs and review sites relevant to the G+ application
- Team at Factspan was able to process approximately 84K Tweets and reviews from social platforms that existed for the brand.
- Build a model considering the n-gram analysis to score our reviews ranging from 0-1. 0 as negative, 0.5 as neutral and 1 as positive
- Perform the sentiment analysis on the score provided by our model to understand the pain points and issues
Interested to read more? Click here!
Sample Reviews & Polarity Scoring:
Polarity Scoring Classification:
Based on approx. 84K reviews that were processed, here are the 3 sentiments calculated
0.0 = Negative
0.5 = Neutral
1.0 = Positive
Tools Used for Whole Analysis:
Following tools were used by the data engineers in Python to perform sentiment analysis for brand product G+
- Stop Word Removal, Lemmatization & Normalization
- N-gram Analysis
- Polarity Scoring
- NLP
- Scrapy
Expected End Results:
Through our predetermined approach, Factspan has been able to and expected to solve the following for brand’s new product G+ newly launched in the market for better product placement.
- High cost savings in revenue
- Lower customer attrition rate by solving for 7 am ride booking issue
- Reduced app crashes due to high traffic at any specific time
- Better working of the recommendation engine in order to serve customers with personalized push notifications at any given time rides are available and park is less crowded
- Attract more customers to sign up for the G+ app and skip the long queue at the physical park location
- Convert the sentiment from negative to positive
A big hurrah to the team involved in this analysis: Ninad Magdum, Azmatul Azam and Sovan Panda
Featured content
Mastering Prompt Engineering Techniques...
A Complete Guide on Security and Complia...
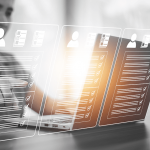
Automating Patient Note Summarization Us...
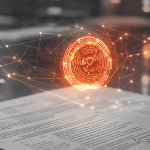
AI Co-Pilot for Smarter Contract Managem...
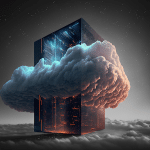
Enhancing Data Processing with Aggregate...
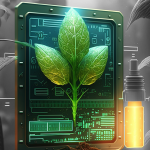
Implementing Sustainable Model Design wi...
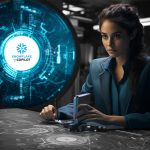
Streamline SQL Workflow with Snowflake C...
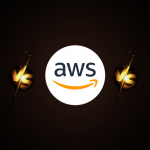