Executive Summary
The client is one of the largest shopping and delivery service providers for local groceries and essential household services in the US. The company has a large pool of independent contractors who will shop and fulfill the orders, placed through a mobile app, for their customers. Due to the vagaries of the business cycle, the client found it difficult to predict the precise availability of the fulfillment partners to deliver the orders on-time to their customers. This caused an imbalance in supply and demand for order fulfillment across multiple cities, thereby impacting the business revenue and customer satisfaction.
To address this challenge, Factspan worked with the client to design and deliver a data analytics model that will help them to better understand the fulfillment partner profile and their likelihood of churn. It will help the client to tailor incentives and retention programs for their active and high-performing segment of fulfillment partners.
About the Client
The client is one of the largest local grocery delivery services in the US and has since expanded to over 200+ cities. Through a membership-based user-friendly app the client connects their customers to a local network of reliable fulfillment and delivery personnel for shopping fresh groceries and everyday essentials.
The fulfillment partners have total control over when they’d work, as well as which parts of their area they’d like to shop. They set their own schedules according to their availability. They shop from the store and deliver groceries and household essentials to customers within a requested time frame. Scheduling, accepting orders, and shopping orders are all done through the fulfillment partner mobile app.
Business Challenge
When the business expanded rapidly, the planning for supply and demand mapping became very challenging for the client. The seasonality of the business cycles added another layer of complexity in predicting the right mapping, incentives, and retention programs for the order fulfillment partners.
The company was losing orders in certain cities as there were not enough fulfillment partners to shop and deliver orders. In other cities, there wasn’t adequate work for the fulfillment partners to keep them engaged. This imbalance prompted them to find answers to below key questions:
1. Which segment of fulfillment partners tend to churn more?
2. Is the churn phenomenon seasonal?
3. Is there a way to improve retention by addressing churn inducing experiences?
Currently, the client’s tech landscape limited them to view the fulfillment partner’s churn only from the point of time-analysis, i.e., the number of personnel active during a selected time. Hence there was a need to have a thorough analysis of the fulfillment partner order acceptance and processing behavior.
Our Solution
The team at Factspan proposed a data analytics model that can help the client to precisely identify the risk of a fulfillment partner not accepting the orders. The team started with defining churn rate and calculating the number of days of activity required to learn a fulfillment partner’s behavior. As a next step, they addressed the problem by building a forecasting (Supervised Machine Learning) model to predict whether an active fulfillment partner gets churned or gets retained in the system.
Once all the key performance indicators (features) are defined and derived from the snowflake database, the team proceeded with the process of data cleaning and preparation, feature engineering and exploratory data analysis, modeling, setting up model run cadence and saving predictions. The solution was highly effective in determining an exhaustive list of key performance indicators which enhanced the prediction model’s capabilities.
Models tested and evaluated against pre-screened criteria: Simple gaussian NB, Simple random test, Simple decision trees, Simple gradient boosting classifier, MLP classifier, XGBoost classifier, and XGBoost classifier with optimized threshold.
Business Impact
- The solution helped the client to design better retention strategies for fulfillment partners and reduce their cost of acquisition
- It helped in better forecasting for hiring, especially during seasonal demands
- It enabled the client to offer tailored incentives to high-valuable fulfillment partners who are at risk of churning
- It further facilitated a process to analyze and develop behavioral models and to better segment partner profiles and their likelihood of churn
- 85%+ accurate prediction of the churn likelihood of a fulfillment partner
Featured content
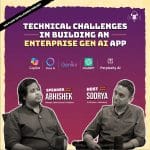
Technical Challenges In Building An Ente...
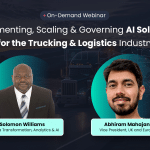
Implementing, Scaling and Governing AI S...
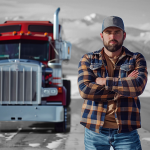
Enhancing CX and Reducing OpEx for Truck...
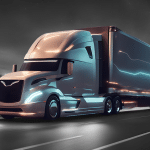
AI-Driven Transformation in Trucking and...
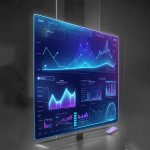